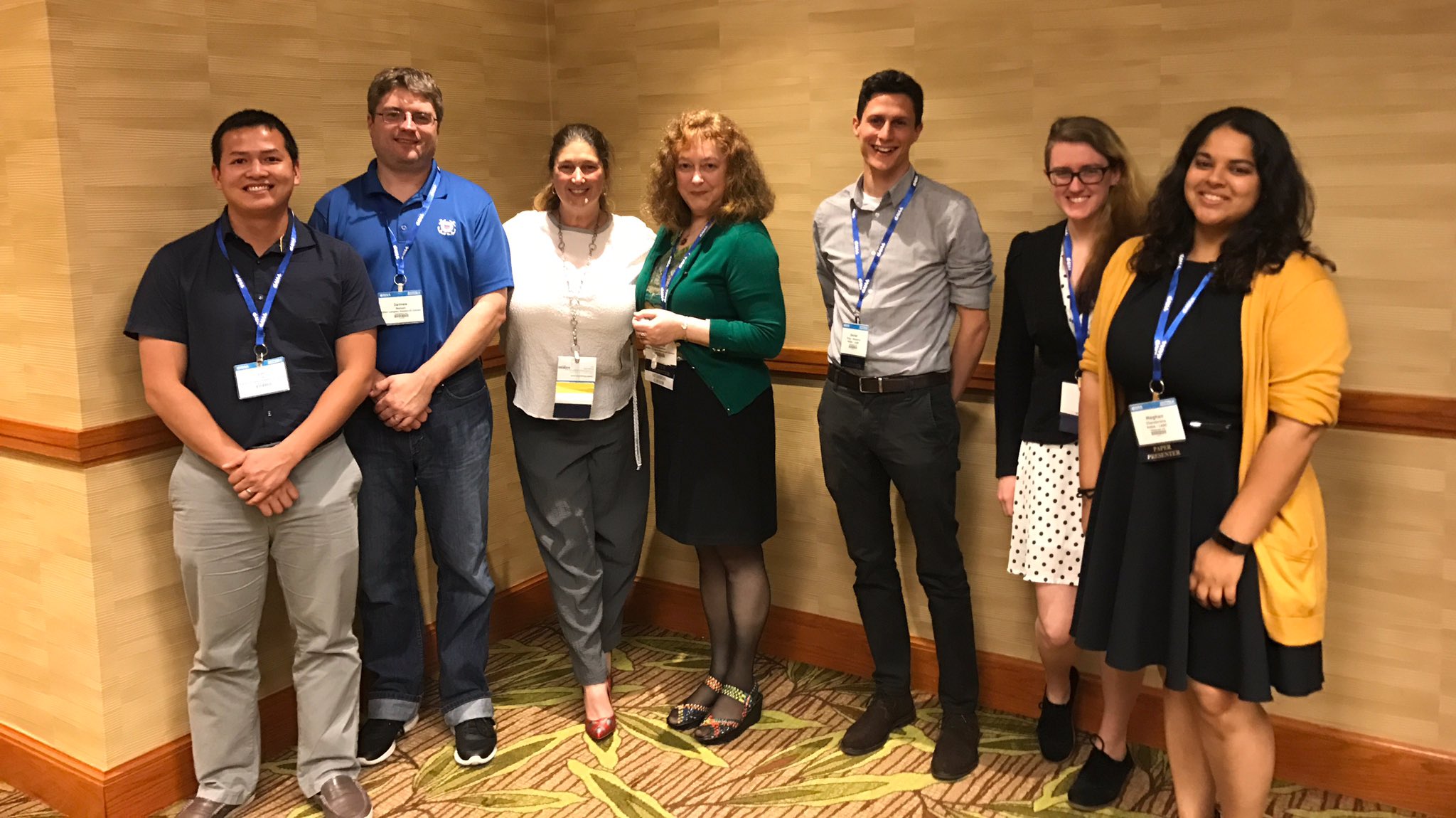 |
The squad celebrates another successful year at AIAA Aviation. |
If AIAA Aviation were the Super Bowl, the Autonomy Incubator would be polishing several rings right now. The success we had in our first special session at
Aviation 2015 led to us receiving even more exposure for our
smash hit presentations at Aviation 2016, and at
Aviation 2017 last week, the hype continued to build-- even if our papers weren't named after Star Trek references this time. Our team presented on the full breadth of the Ai's research, from human-machine interaction to time-coordinated flight, and dominated Wednesday's special session on autonomy.
Dr. Danette Allen, Ai head and NASA Senior Technologist for Intelligent Flight Systems, gave the Ai's first presentation of the week on Wednesday morning during the Forum 360 panel on Human-Machine Interaction (HMI). She presented on ATTRACTOR, a new start that leverages
HOLII GRAILLE (the Ai's comprehensive mission pipeline for autonomous multi-vehicle missions) and seeks to build a basis for certification of autonomous systems with trust and trustworthiness via explainable AI (XAI) and and natural human-machine interaction. Given the proposed emphasis on human-machine interaction HMI and "trusted" autonomy, we are excited to begin working ATTRACTOR (
Autonomy Teaming & TRAjectories
for Complex Trusted Operational Reliability) in October 2017.
 |
Danette Allen at Aviation 2017 |
That afternoon brought the special session on autonomy, which featured a roster of seven NASA Langley scientists-- most of whom were members, alumni, or somehow affiliated with the Ai. Danette opened the session with an overview of the Ai science mission. Jim Nielan followed with a discussion of visual odometry (VO) use in the field. Look
here for examples of Jim testing one of our implementations of VO in the field.
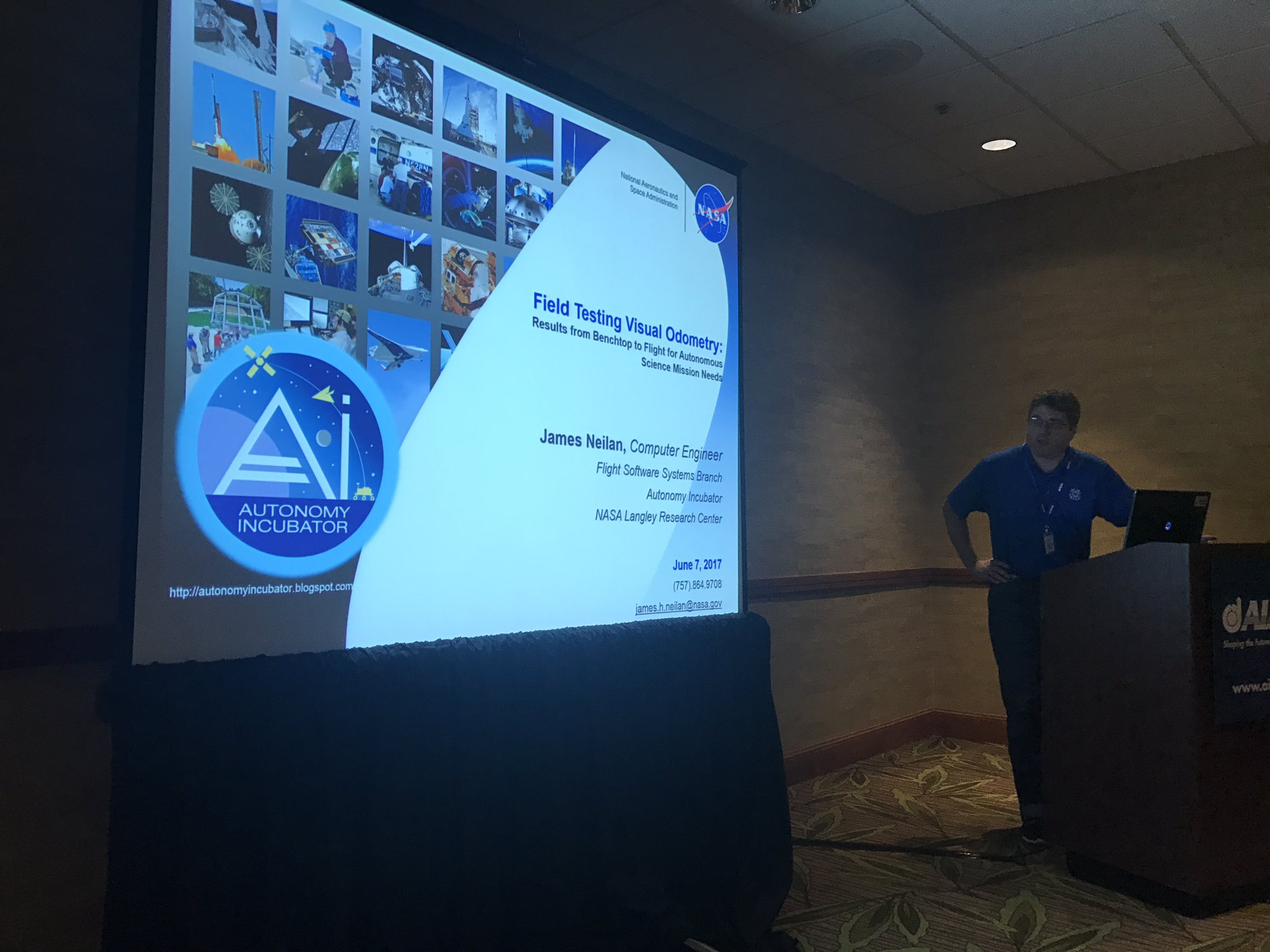 |
Jim Nielan |
Next up, Loc Tran presented on his computer vision work as it applies to precision landing and package detection for autonomous UAVs. Loc's other computer vision work has focused on obstacle avoidance for vehicles flying under the forest canopy, which we affectionately call "
tree-dodging." This summer will hold some exciting updates from his
collaboration with MIT, so stay tuned!
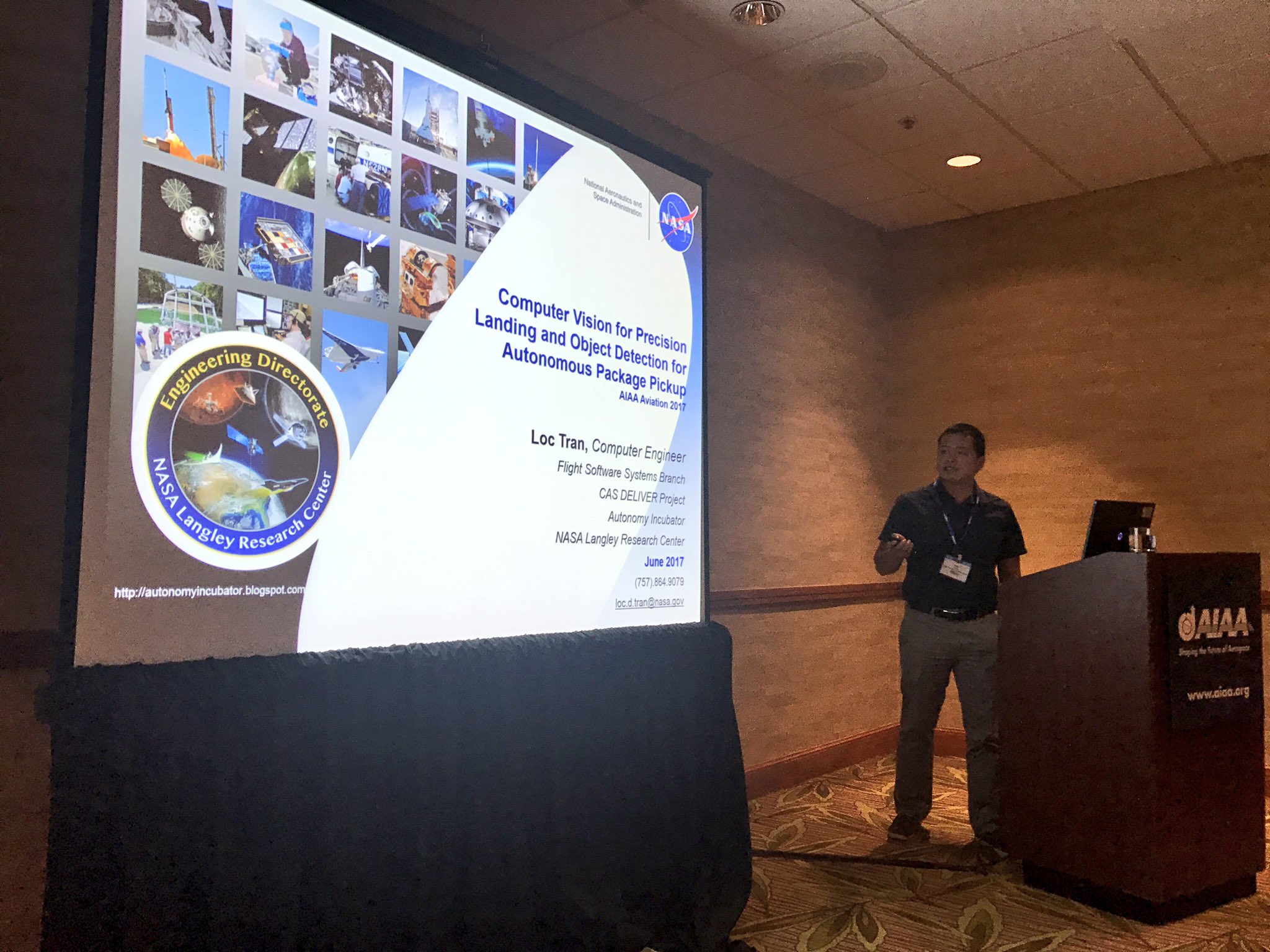 |
Loc Tran |
Javier Puig-Navarro, a PhD candidate at University of Illinois Urbana-Champaign (UIUC) and summer fixture at the Ai, followed with his work on time-coordinated flight. We did an in-depth profile on Javier's work
here on the blog last summer, which will not only give you an idea of what he's doing but also let you see what a delightful person he is.
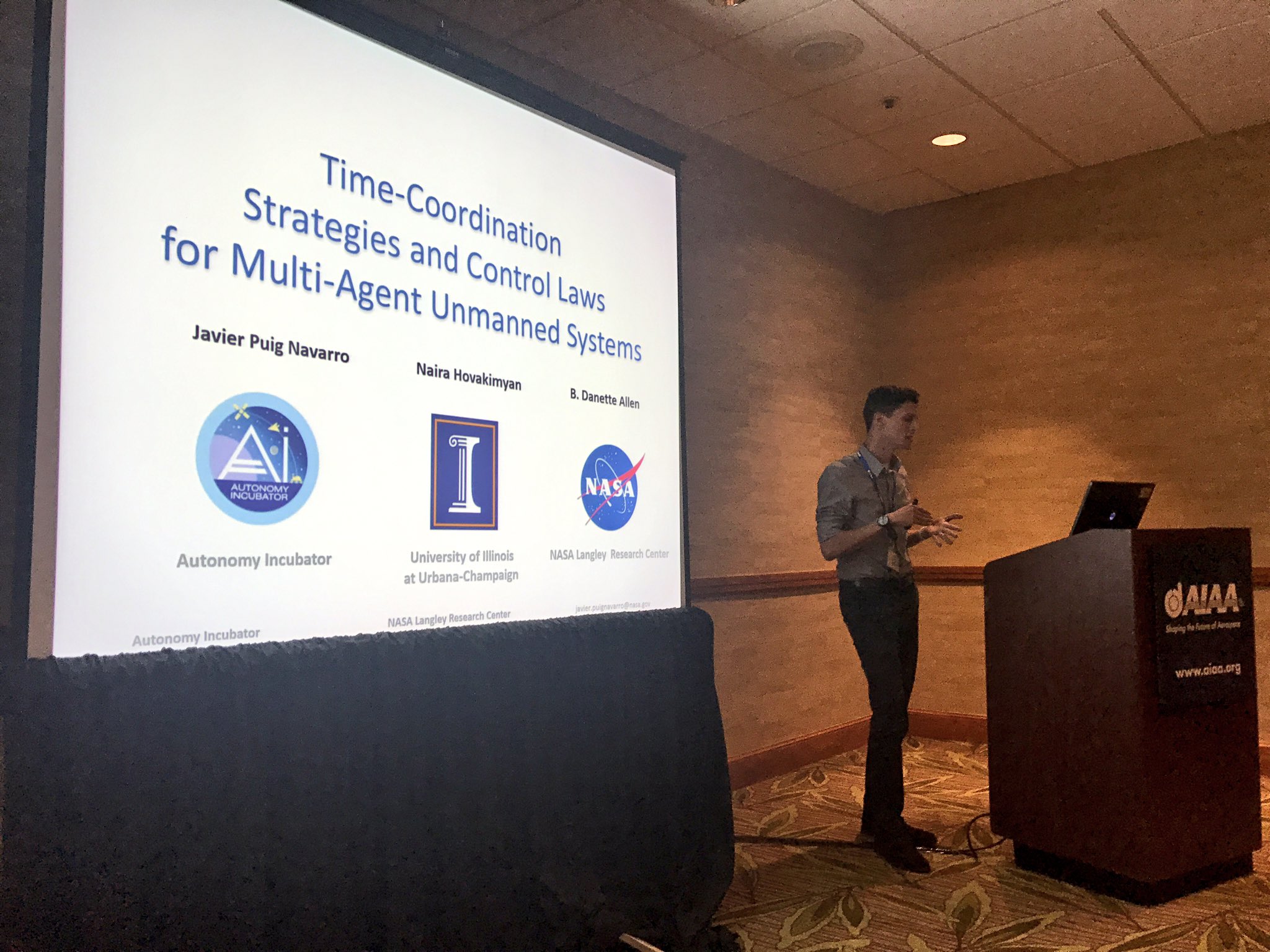 |
Javier Puig-Navarro |
Fellow intern and PhD candidate at Carnegie-Mellon University (CMU), Meghan Chandarana, was up next, talking about gesture recognition as a way to set and adjust flight paths for autonomous UAVs. Meghan's work in gesture controls is as innovative as it is fun to watch, and can be seen in action in the
HOLII GRAILLE demo video from last summer.
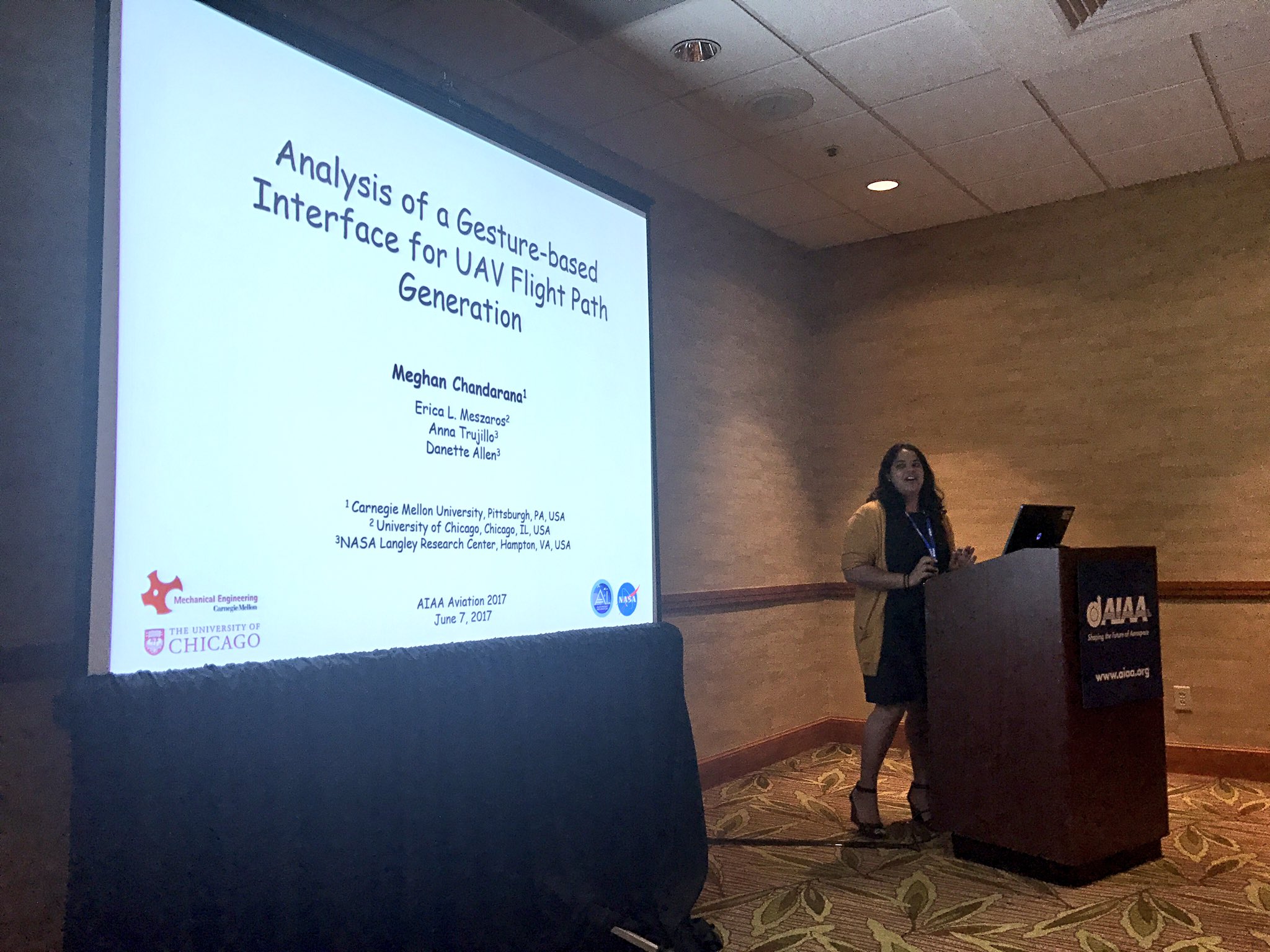 |
Meghan Chandarana |
Erica Meszaros, a former intern pursuing her second Master's degree at the University of Chicago, followed Meghan with a presentation about her amazing natural-language interface between humans and autonomous UAVs. As you know if you've been reading this blog, Erica and Meghan have worked closely to combine their research into a multi-modal interface for generating UAV trajectories. If you're curious about the results of their joint work, we recommend watching their
exit presentation from last summer.
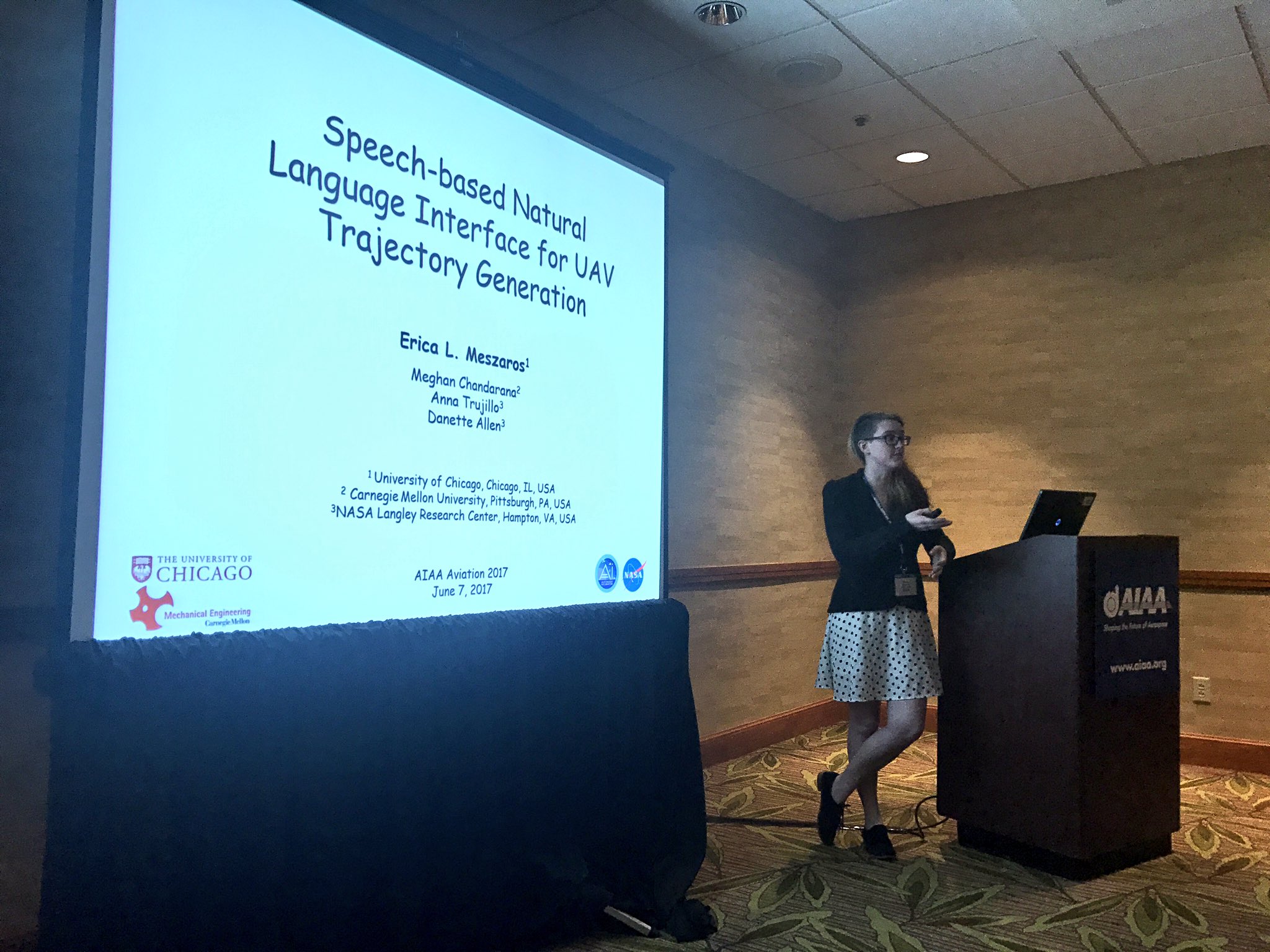 |
Erica Meszaros |
Finally, NASA Langley's own Natalia Alexandrov wrapped up the special session with a presentation on "Explainable AI", an important facet of building trust in human-machine interactions. Natalia and Danette are co-PIs on the ATTRACTOR project, and it was a privilege to have Natalia point to the future and close out the special session on autonomy.
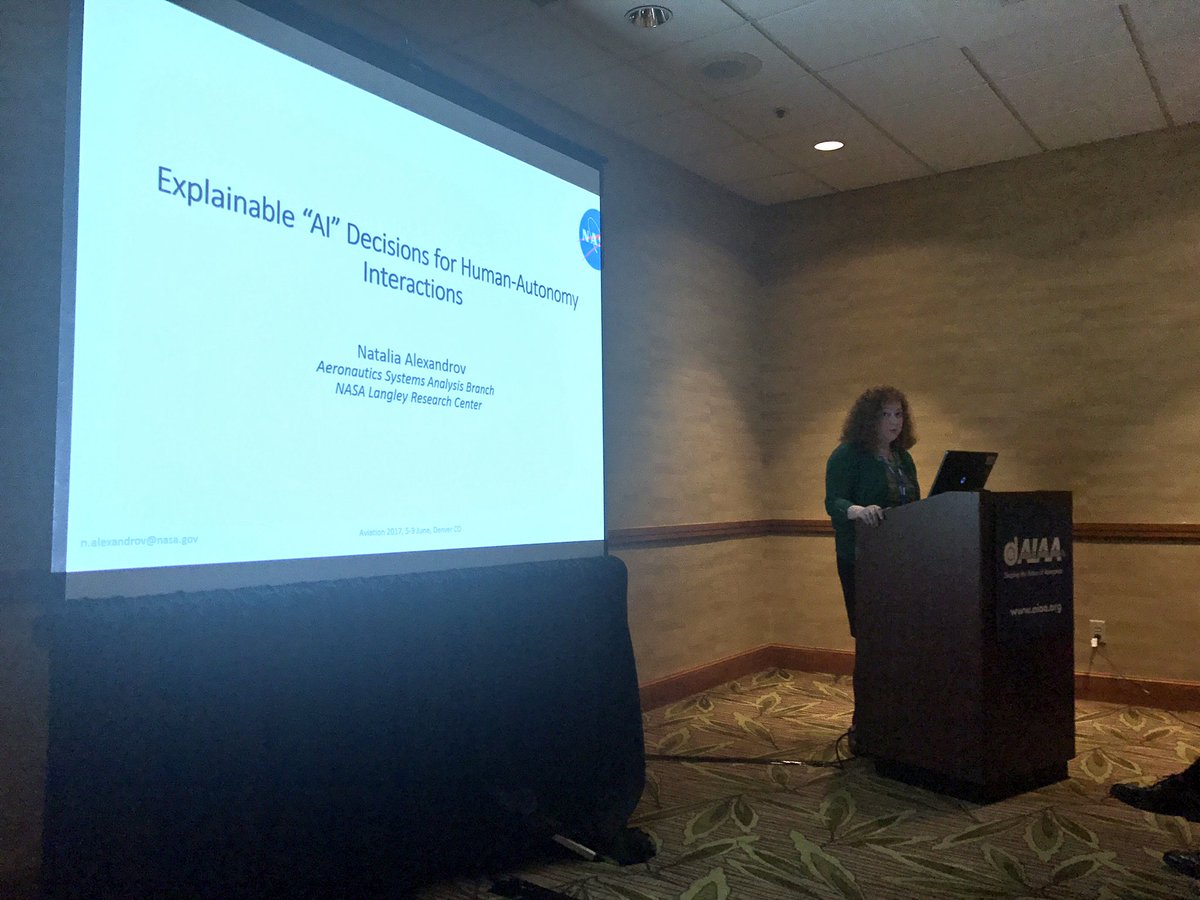 |
Natalia Alexandrov |
Crushing it so hard at Aviation is the perfect way to kick off a summer full of fast-paced, groundbreaking science. Make sure to follow us not just here on the blog, but on
Twitter,
Instagram, and
Facebook as well– you don't want to miss anything, do you?